In this blog, I will show you how to interface an Atari-style joystick to the Altera DE1 FPGA board running a Spectrum implementation, how to change the ROM to enable you to input some game-cheat pokes, and a few games I eventually completed using this setup.
Category: Hacks
Various hacks from all over the map.
Weather Station
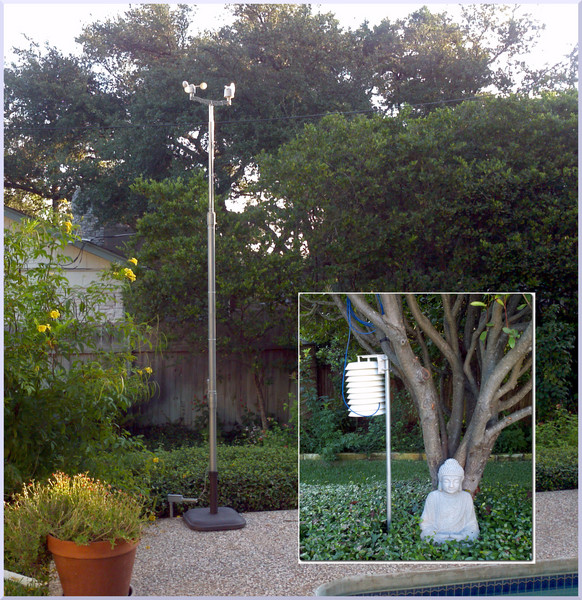
I am using an off-the-shelf anemometer, wind vane, and rain gauge, which I connected to a custom-built ESP32 board that does the data collection and processing before sending it to the home server (a Rock64 SoC with attached SSD). The server software consists of CumulusMX, Emoncms, and various Python files to glue all the pieces together.
The firmware on the ESP32 follows NOAA requirements regarding weather collection observing standards.
The weather data is sent to WeatherCloud.
Every 15 minutes, the data is also uploaded to this website. It partially shows within the frame below; click here to open it in a new tab.
The Anatomy of a Z80 Gate
The data bus on the Z80 processor is 8 bits wide. Data bus wires carrying information within the chip itself do not simply connect to package pins and out to the world – the gate circuitry of each bit is quite complex. This article presents a transistor-level schematic of a data bit’s gate which I reverse-engineered from a die photograph.
Data pins (D0-D7) carry arguably the most complex signals on the Z80 since they are both bi-directional and capable of tri-stating. They are located around the +5V pin – four of them on each side. This is a microphotograph of a gate of one of the data pins which we will look at more closely today – a pin for a data line D6.
Z80 Instruction Register deciphered
After reading excellent Ken Shirriff’s blog on reverse-engineering parts of the Z80 CPU, I decided to learn how to decipher some of the chip die-shots myself. It turns out not to be that difficult if you follow certain guidelines, which I will describe in this post.
Start with a good and clean die shot. Although the Visual 6502 team had a good one, it was somewhat grainy, and I’ve found a much cleaner version here. There is a slight difference in masks, but the functions are the same. In fact, it may even help to look at several versions when trying to decipher the layout.
ZiLOG Z80 (un)documented behavior
In my previous post, I described an Arduino dongle and the software that can be used to clock a Z80 CPU and dump the states of its buses and pins while executing a controlled set of test cases.
Here I show a trace of every single Z80 instruction as run by that setup. I also outlined some of the tests created manually that clarified a few situations which were not too obvious (to me) after reading various pieces of documentation.
Continue readingArduino and ZiLOG Z80
If you want to find out exactly what a venerable Z80 is doing on its bus while executing instructions, in this post I outlined a dongle and the software that will let you see that. Using just a few components and connecting them to an Arduino Mega, you can trace instructions clock by clock and observe what’s happening on the bus.
Printing from Eagle
When printing from Eagle (a PCB CAD software), you can print only one layout per page. Usually, I need two since when I print to transparency, I like to overlap two identical images to get a better “black” for photo-etching.
Using this method I can compose several layouts into a single page to be printed. This also results in less waste.
Parallel Port Logic Analyzer
A few months ago I had ordered a new PCI parallel port card. It turned out my computer did not have a parallel port and I wanted to try the parallel port logic analyzer software described here:
http://www.codeproject.com/Articles/75279/17-Channel-Logic-Analyzer
Software Scope using a Sound Card
I tried a simple software implementation of a digital oscilloscope using a PCI sound card and this software: http://www.zeitnitz.de/Christian/scope?mid=2
I connected its own signal generator (from the line out) into the line in and it worked — sort of. The input capacitors were skewing low frequencies. I de-soldered those caps but then hit a bigger problem of a floating DC component. Also, there was a lot of noise on the line.
Ambient Weather WS-2080 hang fix
A weather station, Ambient Weather WS-2080 that I installed in my backyard has a receiver unit with a pretty nasty hardware issue: occasionally, its USB interface would hang hard. It could happen twice a week or once a month – it is very unpredictable and highly annoying. Once it happens, nothing short of a power reset of the receiver unit would fix it. You’d have to remove the battery or unplug the USB if you powered it through it. I’ve finally got around to fixing it. If you have a similar problem with that weather station, you may be interested to read on…